Deep Learning in Practice: A Comprehensive Guide to Real-World Applications

Deep learning is a subfield of machine learning that has gained significant attention in recent years due to its ability to solve complex problems that were previously intractable. Deep learning models are able to learn from large amounts of data and extract complex patterns, making them well-suited for tasks such as image recognition, natural language processing, and speech recognition.
In this article, we will provide a comprehensive overview of deep learning in practice. We will discuss the different types of deep learning models, their applications in various industries, and the potential benefits and challenges of using deep learning for real-world problems.
There are many different types of deep learning models, each with its own strengths and weaknesses. Some of the most common types of deep learning models include:
4.6 out of 5
Language | : | English |
File size | : | 14824 KB |
Print length | : | 198 pages |
Paperback | : | 77 pages |
Item Weight | : | 5.4 ounces |
Dimensions | : | 5.5 x 0.19 x 8.5 inches |
Screen Reader | : | Supported |
- Convolutional Neural Networks (CNNs) are designed to process data that has a grid-like structure, such as images. CNNs are commonly used for tasks such as image classification, object detection, and facial recognition.
- Recurrent Neural Networks (RNNs) are designed to process sequential data, such as text and speech. RNNs are commonly used for tasks such as natural language processing, speech recognition, and machine translation.
- Transformer Neural Networks are a newer type of deep learning model that has shown promising results on a variety of tasks, including natural language processing, computer vision, and speech recognition. Transformers are similar to RNNs, but they use a different attention mechanism that allows them to learn long-term dependencies in the data.
Deep learning has a wide range of applications in various industries, including:
- Healthcare: Deep learning is being used to develop new methods for diagnosing diseases, predicting patient outcomes, and developing new drugs. For example, deep learning models have been used to detect skin cancer with the same accuracy as dermatologists, and to predict the risk of heart disease with high accuracy.
- Finance: Deep learning is being used to develop new methods for fraud detection, risk assessment, and trading. For example, deep learning models have been used to detect fraudulent transactions with high accuracy, and to predict stock market movements with some success.
- Manufacturing: Deep learning is being used to develop new methods for quality control, predictive maintenance, and process optimization. For example, deep learning models have been used to detect defects in manufactured products with high accuracy, and to predict when equipment is likely to fail.
- Transportation: Deep learning is being used to develop new methods for self-driving cars, traffic management, and logistics. For example, deep learning models have been used to develop self-driving cars that can navigate complex road conditions with high accuracy, and to optimize traffic flow in real-time.
There are many potential benefits to using deep learning for real-world problems, including:
- Accuracy: Deep learning models can achieve high levels of accuracy on complex tasks, even when the data is noisy or incomplete.
- Generalizability: Deep learning models can generalize well to new data, even if the new data is different from the data that the model was trained on.
- Efficiency: Deep learning models can be trained on large amounts of data in a relatively short amount of time.
- Automation: Deep learning models can be used to automate tasks that would otherwise require human intervention.
There are also some challenges associated with using deep learning for real-world problems, including:
- Data requirements: Deep learning models require large amounts of data to train, which can be difficult to collect and label.
- Computational requirements: Deep learning models can be computationally expensive to train, which can require specialized hardware such as GPUs.
- Interpretability: Deep learning models can be difficult to interpret, which can make it challenging to understand how they make decisions.
- Bias: Deep learning models can be biased, which can lead to unfair or inaccurate decisions.
Deep learning is a powerful tool that has the potential to solve complex problems that were previously intractable. However, it is important to be aware of the challenges associated with using deep learning, such as the data requirements, computational requirements, interpretability issues, and bias. By carefully considering the benefits and challenges of deep learning, organizations can make informed decisions about whether or not to use deep learning for their specific needs.
As deep learning continues to develop, we can expect to see even more innovative applications of this technology in the years to come. Deep learning has the potential to revolutionize a wide range of industries, and it is important to be prepared for the impact that this technology will have on our world.
4.6 out of 5
Language | : | English |
File size | : | 14824 KB |
Print length | : | 198 pages |
Paperback | : | 77 pages |
Item Weight | : | 5.4 ounces |
Dimensions | : | 5.5 x 0.19 x 8.5 inches |
Screen Reader | : | Supported |
Do you want to contribute by writing guest posts on this blog?
Please contact us and send us a resume of previous articles that you have written.
Text
Story
Genre
Paperback
E-book
Magazine
Newspaper
Sentence
Bookmark
Shelf
Glossary
Bibliography
Preface
Manuscript
Scroll
Codex
Bestseller
Classics
Autobiography
Encyclopedia
Thesaurus
Narrator
Character
Resolution
Librarian
Catalog
Research
Lending
Reserve
Academic
Journals
Reading Room
Rare Books
Literacy
Study Group
Dissertation
Storytelling
Awards
Book Club
Textbooks
Donna Shea
Ernie Jackson
Dejan Sarka
Bob Orrell
Brian Requarth
Jemma Spark
Charles Mccarry
Willis H Thomas
Ciaran Nagle
Jamie Markle
Jeffrey Lant
Connor Hoover
Thomas Taylor
Braeden Michaels
Heidi Pitlor
Tina Stoecklin
Brian Green
John Dunlavey
Stephen Brooks
Kristiana Sfirlea
Light bulbAdvertise smarter! Our strategic ad space ensures maximum exposure. Reserve your spot today!
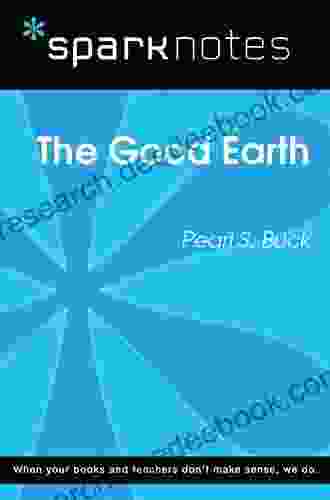

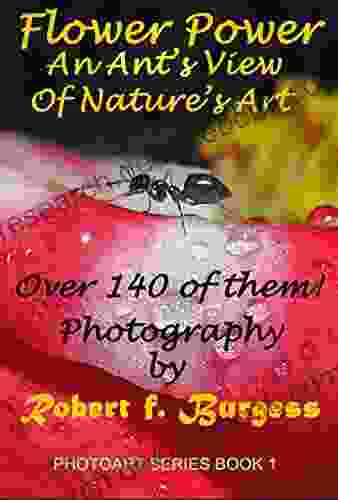

- Ernest J. GainesFollow ·7.2k
- Charlie ScottFollow ·8.7k
- Simon MitchellFollow ·13.4k
- Joe SimmonsFollow ·5.3k
- Fernando PessoaFollow ·6.4k
- Ted SimmonsFollow ·3k
- Christian BarnesFollow ·6.6k
- Mike HayesFollow ·6.7k
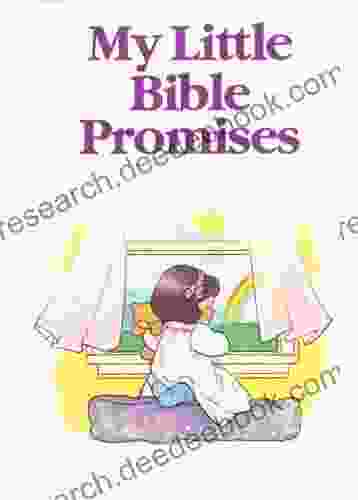

My Little Bible Promises Thomas Nelson
In a world filled with uncertainty and...
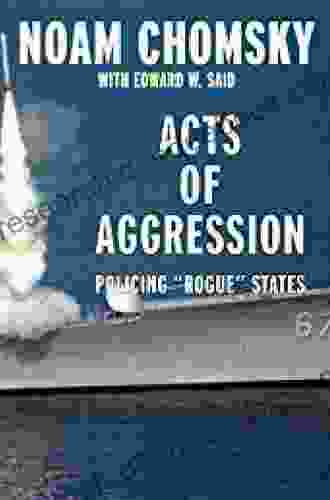

Policing Rogue States: Open Media Series Explores Global...
In today's interconnected...
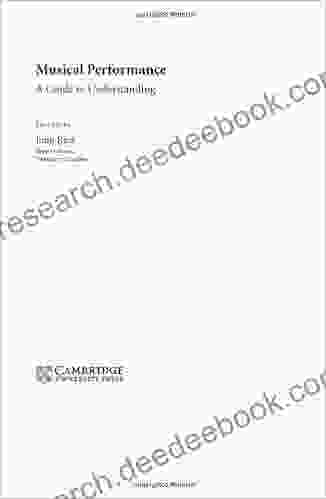

Musical Performance: A Comprehensive Guide to...
Immerse yourself in the...
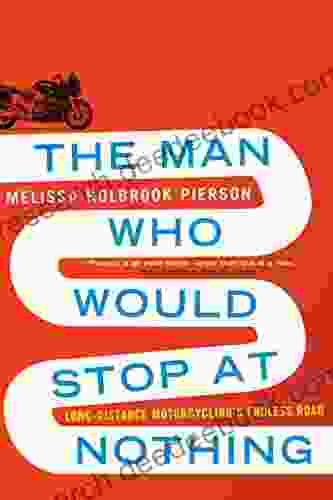

Long Distance Motorcycling: The Endless Road and Its...
For many, the...
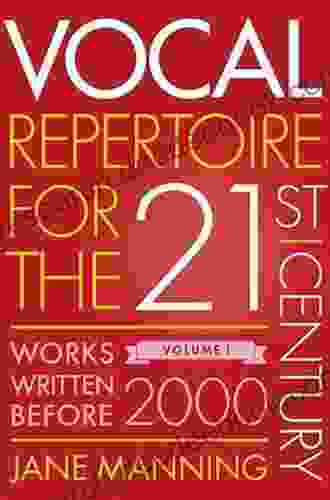

Vocal Repertoire for the Twenty-First Century: A...
The vocal repertoire of the twenty-first...
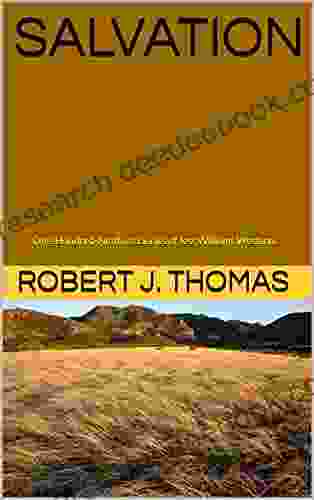

One Hundred and Ninth on the Call Sheet! The Enigmatic...
In the vast panorama of Western films,...
4.6 out of 5
Language | : | English |
File size | : | 14824 KB |
Print length | : | 198 pages |
Paperback | : | 77 pages |
Item Weight | : | 5.4 ounces |
Dimensions | : | 5.5 x 0.19 x 8.5 inches |
Screen Reader | : | Supported |